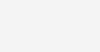
Oct 31 2023
/
Implementing Predictive Maintenance for Industrial Machinery
Predictive maintenance is a proactive approach to equipment maintenance that uses data analysis to predict when failures are likely to occur. By analyzing data from sensors and other sources, machine learning algorithms can identify patterns that indicate when a component is expected to fail. This allows businesses to schedule maintenance tasks before failures occur, minimizing downtime and disruption to operations.
Predictive maintenance is particularly beneficial for industrial machinery, which is often critical to the manufacturing process. Unplanned downtime of industrial machinery can lead to significant losses in productivity and revenue. Predictive maintenance can help to avoid these losses by ensuring that equipment is always in good working order.
Benefits of Predictive Maintenance
Predictive maintenance offers a number of benefits for businesses, including:
- Reduced downtime: Predictive maintenance can help to reduce downtime by identifying and addressing potential problems before they cause a failure. This can lead to significant savings in terms of lost productivity and revenue.
- Increased productivity: By reducing downtime, predictive maintenance can help to increase productivity. Additionally, predictive maintenance can help to improve the quality of products by preventing defects caused by equipment failures.
- Improved asset reliability: Predictive maintenance can help to improve the reliability of assets by identifying and addressing potential problems before they cause a failure. This can lead to extended asset life and reduced maintenance costs
- Reduced maintenance costs: Predictive maintenance can help to reduce maintenance costs by avoiding the need for unplanned repairs and replacements. Additionally, predictive maintenance can help to optimize maintenance schedules, leading to more efficient use of maintenance resources.
- Improved safety: By identifying and addressing potential problems before they cause a failure, predictive maintenance can help to improve safety in the workplace. This is especially important in industries where equipment failures can have serious consequences for safety, such as manufacturing and transportation.
- Reduced environmental impact: By reducing unplanned equipment failures, predictive maintenance can help to reduce the environmental impact of businesses. This is because unplanned equipment failures can often lead to the release of pollutants into the environment.
How Predictive Maintenance Works
Predictive maintenance works by analyzing data from sensors and other sources to identify patterns that indicate when a component is expected to fail. This data can be collected using a variety of methods, including:
- Condition monitoring sensors: Condition monitoring sensors can be used to collect data on a variety of parameters, such as vibration, temperature, and pressure. This data can be used to identify changes in the condition of a machine that may indicate a potential problem.
- Historical maintenance records: Historical maintenance records can be used to identify patterns in equipment failures. This information can then be used to predict when similar failures are likely to occur in the future.
- Operational data: Operational data, such as production data and energy consumption data, can also be used for predictive maintenance. This data can be used to identify changes in the operation of a machine that may indicate a potential problem.
Once the data has been collected, it is analyzed using machine learning algorithms to identify patterns that indicate impending failure. The machine learning algorithms can be trained on historical data or on data that is collected from a fleet of machines.
Once the machine learning model has been trained, it can be used to predict the probability of failure for any given machine at any given time. This information can then be used to schedule maintenance tasks before failures occur.
Implementing Predictive Maintenance
There are a number of steps involved in implementing predictive maintenance for industrial machinery:
- Identify critical assets: The first step is to identify the most critical assets in the manufacturing process. These are the assets that would cause the most disruption to operations if they were to fail.
- Collect data: Once the critical assets have been identified, it is necessary to collect data on their performance. This data can be collected using a variety of methods, such as condition monitoring sensors, historical maintenance records, and operational data.
- Clean and prepare the data: Once the data has been collected, it needs to be cleaned and prepared for analysis. This involves removing any errors or inconsistencies in the data and transforming it into a format that can be used by the machine learning algorithms.
- Select and train a machine learning model: There are a number of different machine learning algorithms that can be used for predictive maintenance. The best algorithm to use will depend on the specific type of data that is available and the desired outcome.
- Deploy the predictive maintenance solution: Once the machine learning model has been trained, it can be deployed to production. This may involve developing a software application or integrating the model into an existing maintenance management system.
- Monitor and refine the model: It is important to monitor the performance of the predictive maintenance model over time and refine it as needed. This can be done by feeding the model new data and observing its predictions.
Here are a few case studies demonstrating how predictive maintenance can save costs and improve equipment reliability:
- GE Aviation uses predictive maintenance to monitor the health of its jet engines. By analyzing data from sensors on the engines, GE can identify potential problems before they cause a failure. This has helped GE to reduce unplanned engine maintenance events by 50% and save the company billions of dollars in annual costs.
- Rolls-Royce uses predictive maintenance to monitor the health of its marine engines. By analyzing data from sensors on the engines, Rolls-Royce can identify potential problems before they cause a failure. This has helped Rolls-Royce to reduce unplanned engine maintenance events by 25% and save the company millions of dollars in annual costs.
- SKF is a manufacturer of bearings and seals. SKF uses predictive maintenance to monitor the health of its bearings in its customers’ equipment. By analyzing data from sensors on the bearings, SKF can identify potential problems before they cause a failure. This has helped SKF’s customers to reduce unplanned downtime by 30% and save millions of dollars in annual costs.
- United Airlines uses predictive maintenance to monitor the health of its aircraft. By analyzing data from sensors on the aircraft, United can identify potential problems before they cause a failure. This has helped United to reduce unplanned aircraft maintenance events by 15% and save the company millions of dollars in annual costs.
These are just a few examples of how predictive maintenance can save costs and improve equipment reliability. Predictive maintenance can be used in a wide variety of industries, including manufacturing, transportation, and utilities.
How to Get Started with Predictive Maintenance
If you are interested in implementing predictive maintenance for your business, there are a few things you can do to get started:
- Identify your critical assets: Which assets are most important to your business and would cause the most disruption if they were to fail?
Collect data on your assets. This data can be collected using a variety of methods, such as condition monitoring sensors, historical maintenance records, and operational data. - Clean and prepare the data: Once the data has been collected, it needs to be cleaned and prepared for analysis. This involves removing any errors or inconsistencies in the data and transforming it into a format that can be used by machine learning algorithms.
- Select and train a machine learning model: There are a number of different machine learning algorithms that can be used for predictive maintenance. The best algorithm to use will depend on the specific type of data that is available and the desired outcome.
- Deploy the predictive maintenance solution: Once the machine learning model has been trained, it can be deployed to production. This may involve developing a software application or integrating the model into an existing maintenance management system.
- Monitor and refine the model: It is important to monitor the performance of the predictive maintenance model over time and refine it as needed. This can be done by feeding the model new data and observing its predictions.
Conclusion
Predictive maintenance is a rapidly growing field with the potential to revolutionize the way we maintain industrial machinery. By using data analysis to predict when failures are likely to occur, predictive maintenance can help businesses to avoid costly downtime and ensure that their equipment is always in good working order.
The benefits of predictive maintenance are clear. By reducing downtime, increasing productivity, improving asset reliability, and reducing maintenance costs, predictive maintenance can help businesses to improve their bottom line. Additionally, predictive maintenance can help to improve safety and reduce the environmental impact of businesses.
Here are some additional thoughts on the conclusion of predictive maintenance:
- Predictive maintenance is still a relatively new technology, but it is rapidly maturing. The increasing availability of sensor data and the development of more powerful machine learning algorithms are making predictive maintenance more feasible and affordable than ever before.
- Predictive maintenance is not a silver bullet. It is important to note that predictive maintenance is not a replacement for preventive maintenance. Predictive maintenance can help to identify and address potential problems before they cause failures, but it is still necessary to perform regular preventive maintenance tasks.
- The success of predictive maintenance depends on the quality of the data. The more data that is available, and the higher the quality of the data, the more accurate the predictions of the predictive maintenance model will be.
Overall, predictive maintenance is a powerful tool that can help businesses to improve their operations, reduce costs, and improve safety.