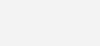
Oct 17 2024
/
AI in Predictive Maintenance for Legacy Systems
As manufacturing industries embrace digital transformation, one of the most significant challenges is maintaining older, legacy systems that are still in use. While these systems have often proven reliable over decades, they can become a bottleneck for innovation and efficiency due to their age and limitations. Enter AI-driven predictive maintenance—an emerging solution that not only extends the life of legacy equipment but also helps manufacturers reduce costly unplanned downtime.
The Challenge of Legacy Systems
Manufacturing environments often contain a mix of cutting-edge technology and decades-old equipment. While upgrading or replacing legacy systems with new, more efficient machinery seems like an obvious choice, it’s not always feasible. High costs, long downtimes for replacements, or the necessity of custom-built solutions keep many manufacturers tied to legacy equipment.
However, these older systems are prone to mechanical failures, leading to unplanned downtime that can disrupt production schedules and cause significant financial losses. Traditional maintenance strategies, such as reactive and preventive maintenance, are no longer adequate to address these challenges. They either wait for breakdowns to occur (reactive) or follow fixed maintenance schedules (preventive), both of which are inefficient and expensive.
How AI-Driven Predictive Maintenance Works
Predictive maintenance leverages the power of AI, machine learning, and data analytics to monitor and predict when equipment will likely fail. This approach collects real-time data from sensors attached to machines and uses advanced algorithms to analyze patterns, detect anomalies, and forecast potential failures before they happen.
Here’s how AI-driven predictive maintenance works:
1. Data Collection
Sensors installed on legacy machines monitor various parameters such as vibration, temperature, noise levels, and pressure. This real-time data is transmitted to a central system for analysis.
2. Data Analysis
AI algorithms, particularly those involving machine learning, process the incoming data, compare it to historical information, and detect abnormal behavior that could indicate an upcoming failure.
3. Prediction and Alerts
When the AI system identifies a potential failure, it sends alerts to the maintenance team. The team can then intervene and schedule repairs during planned downtime, minimizing disruption to production.
4. Continuous Improvement:
AI models improve over time as they learn more about the machine’s operational patterns. The more data they collect, the more accurate their predictions become.
Benefits of Predictive Maintenance for Legacy Systems
1. Extended Equipment Life
With AI-driven predictive maintenance, manufacturers can prolong the life of their legacy systems by identifying potential issues early and preventing catastrophic failures. This reduces the need for expensive, full-scale replacements while allowing equipment to operate more efficiently.
2. Reduced Downtime
One of the most significant advantages is the reduction of unplanned downtime. Traditional maintenance methods often result in either too much maintenance (preventive) or none at all until a breakdown occurs (reactive). Predictive maintenance ensures that repairs are only done when truly necessary, minimizing interruptions to production.
3. Lower Maintenance Costs
Predictive maintenance helps optimize the use of spare parts and labor. Instead of over-maintaining machines or waiting until breakdowns cause more significant problems, AI systems predict when specific components will wear out. This allows for targeted, cost-effective interventions that reduce both material waste and labor hours.
4. Improved Safety
Equipment failures can sometimes pose safety risks to workers on the shop floor. By identifying and addressing issues before they escalate into critical failures, predictive maintenance enhances workplace safety.
5. Better Resource Allocation
AI-driven predictive maintenance provides manufacturers with data-driven insights that enable better planning and resource allocation. With predictive models in place, maintenance teams can focus their efforts on the equipment most at risk of failure, ensuring that resources are used efficiently.
Overcoming Integration Challenges
Integrating AI predictive maintenance with legacy systems poses some challenges. Older equipment may not be equipped with the necessary sensors or connectivity for data collection. However, many manufacturers are overcoming these challenges by retrofitting their machines with IoT sensors, allowing for real-time data capture and connectivity.
Additionally, AI systems require large amounts of data to function accurately. Initially, legacy machines may not provide enough historical data to create accurate predictions. However, over time, as more data is gathered, AI models become increasingly reliable.
The Future of AI in Manufacturing
As AI and IoT technologies evolve, predictive maintenance is expected to become a standard practice for managing both new and legacy systems. The rise of cloud computing and edge computing also enables more accessible and scalable AI solutions, allowing manufacturers of all sizes to benefit from this technology.
For manufacturers relying on legacy systems, AI-driven predictive maintenance is a game-changer. By using predictive models to foresee equipment failures before they happen, companies can extend the life of aging machinery, reduce unplanned downtime, lower costs, and improve overall operational efficiency. In an era where productivity and uptime are critical to staying competitive, this proactive maintenance approach is key to bridging the gap between legacy systems and modern-day manufacturing needs.
Conclusion
The integration of AI into predictive maintenance marks a significant advancement in managing legacy systems. Manufacturers no longer need to choose between the high cost of replacing older equipment and the inefficiencies of traditional maintenance methods. Instead, AI offers a sustainable, data-driven solution that ensures the longevity of legacy systems while enhancing overall performance. As technology continues to progress, predictive maintenance will undoubtedly play a crucial role in the future of manufacturing, transforming the way businesses maintain and optimize their operations.